Fixes That Fail
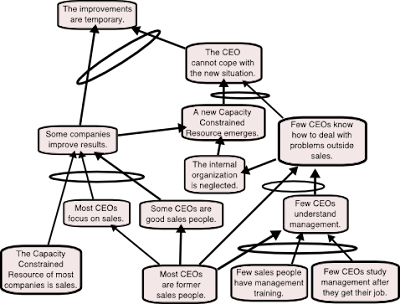
Many companies use a standard response in troubled times, they appoint a new CEO. The new CEO takes measures. If the CEO is a former sales person, he focuses on improving sales. If the CEO is a former accountant, there will be a savings program. A CEO with a technical background will focus on developing new products. The outcome is usually one of the following: The company takes a nosedive, crashes and burns. Nothing happens. The gradual decline continues. Eventually yet another CEO is appointed. There is improvement. Sometimes the improvement is radical. after awhile, the rate of improvement abates. Then the company begins to backslide. Eventually another CEO is appointed. There is a sustained improvement in the company's financial health. This is rare though. The third alternative, initial success followed by backsliding, is in many ways the most interesting outcome. First of all it is a common outcome. Second, it often seems inexplicable. The CEO proved his management genius